AI has been the hottest topic in tech for a bit now and no stranger to the UX research field — 77% of respondents to our AI in UXR survey said that they’re using AI in some part of their work.
As conversations on AI and its impact on UXR continue to grow and evolve, I wanted to share how we see the technology affecting different parts of the UXR stack and how we are using it at User Interviews.
I’ll give a spoiler on the takeaway for User Interviews—we’ve intentionally chosen to focus on using AI to provide real value, which in our case means powering our audience matching behind the scenes. Our advances in AI-powered recruitment are allowing us to find more niche participants, faster, with a lower fraud rate, which will help all people do research and discover insights faster.
All the above said, I’ve seen AI rapidly evolving and wanted to share a few observations on how User Interviews see AI’s impact on the UX research landscape.
Editor’s note: When discussing AI in this article, I am primarily referencing generative AI powered by large language models (LLMs).
Explore the AI tools landscape in our latest UX Tools Map.
The AI Tools Landscape
Looking at the broader market, we see that there are a massive amount of UXR tools, but I’ll simplify by saying you need a tool to:
- Conduct your research method of choice
- Collect and store the insights from the research
- Recruit or manage participants

We’re seeing AI implemented across all three of these areas, which I’ll talk about in greater detail below.
Tools for conducting research tests
Lightweight AI features
Many companies are releasing lightweight AI features utilizing LLMs. These are similar to AI features we’re seeing across other SaaS tools like type-aheads, analysis, and automated summaries. These definitely add some value (for example I’m seeing a lot of researchers use automated summaries of their sessions), however I don’t consider them a step-wise change for researchers.
AI-moderation tools
There is also a new crop of AI-native startups trying to disrupt this layer of the stack. Companies like Heard, Listen Labs, Outset, ResearchGOAT, and more are building tools where an AI agent will moderate the study instead of a human moderator. They are claiming that this allows companies to do more research faster, for example Outset’s headline banner is “The scale of a survey. The depth of an interview.”
These tools are all early and it’s yet to be seen how the market will respond. That said, there is anecdotal evidence that there is a place for them in the market. For example, I came across this post on LinkedIn where Andrea Knight Dolan did an experiment and her observation was that Outset did a better job than some interns and junior UX researchers she had observed doing research.
You can see an example of the output from these tools in this Listen Labs case study where they used their tool to interview 60 users of GLP-1 drugs and then write the report.
Insight repositories
Insight repositories allow companies to store and share the output from research, and then reuse the insights.
I believe this use case is perfect for AI and LLMs. The companies in this space, like Dovetail and Marvin, agree and are investing heavily in AI. These products ingest a lot of qualitative data and then easily allow their users to organize, summarize, and refer back to this data. Before LLMs this was done though complicated labeling, filtering, and organization structures.
LLMs should allow users to use text interfaces and natural language to easily sift through previous research their company has done and stand to make the manual effort of categorizing insights obsolete over time.
Watch Now: UX Research & Artificial Intelligence: Balancing Hype with Humanity
Participant recruitment and management tools
This layer of the stack is obviously where User Interviews has been building.
Synthetic users
So far there have been some startups trying to provide synthetic users. These tools use AI bots to replace participants (as opposed to the AI-moderation tools which are using them to replace moderators). My current read on these tools is that the market is very pessimistic about them. For example, Kate Moran and Maria Rosala from NN/g wrote this great report, with the takeaway being Synthetic users are fake users generated by AI.
While there may be a few use cases for them, we believe user research without human users isn’t user research.
Additionally, I believe this concept misunderstands both how LLMs work and how research works. For example, there should not be existing training data that would allow someone to do a usability test on a new design. I admit I am likely biased here, so I am open to other perspectives!
User Interviews and AI
At User Interviews, we’re laser-focused on helping companies find real, human participants for research studies.
The biggest investment we are making is using AI to help us better match participants to studies. We have a rich proprietary dataset (hundreds of millions of data points) built through almost a decade of recruiting and screening participants. We are layering AI on top of this dataset to better predict which participant will qualify for which studies, based off all the information that participant has previously shared with us. We have a second model that is using all of those data points to help detect fraud. These advances in AI-powered recruitment are allowing us to find more niche participants, faster, with a lower fraud rate.
We’re excited to be the first and only AI-powered recruitment platform in the industry.
This is behind the scenes. If you never notice the AI itself, but have a great experience because of it, we consider that a win. We explicitly chose to focus on implementing AI in ways that provide real value to both researchers and participants, instead of just building a marketing gimmick. The initial investments we are making are having huge effects with participant retention and our ability to fill projects. We’re excited to be the first and only AI-powered recruitment platform in the industry.
I’d love to hear how the rest of the industry is using AI, what tools have been helpful, and any ideas for how AI can help researchers and companies discover and implement qualitative insights.
Let me know your thoughts on LinkedIn.
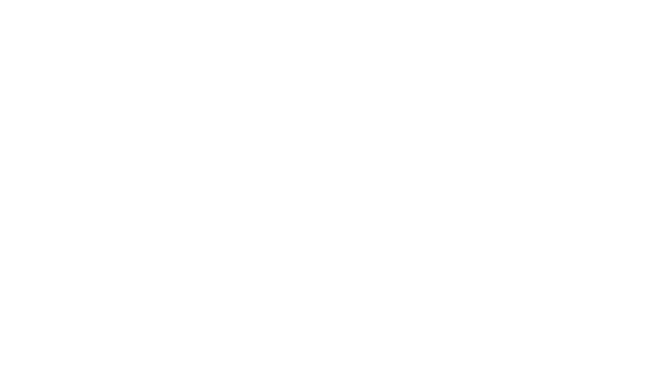