Most of the time, when we ask “what is a good sample size for qualitative research?,” what we are really asking is:
“How many participants do I need to reach saturation?”
Another way of asking that is:
“How many participants do I need to feel confident in the insights I present to my stakeholders or clients?”
For the uninitiated, this might seem like a fairly straightforward question to answer. But anyone that has had to justify qualitative study sizes to stakeholders will know that the “optimal” qualitative sample size is a tough nut to crack.
The good news is that I may have kinda maybe sorta done it?
At the very least, I’ve created a formula for estimating the sample size you need to reach saturation for 7 different qualitative methods—and I feel pretty good about its application in User Research.
Do I recommend that clinical researchers conducting studies with life-or-death consequences rely on this formula? Please no.
Do I feel confident that these recommendations are supported by empirical data, industry best practices, peer-reviewed studies, and are made with consideration for the realities of doing research as part of a product development cycle? Yes—and after reading this article, hopefully you will too.
👉 You can see this formula in action and read a bit about the methodology behind it by heading to our new Qualitative Sample Size Calculator.
A formula for determining qualitative sample size
In 2013, Research by Design published a whitepaper by Donna Bonde which included research-backed guidelines for qualitative sampling in a market research context.
Victor Yocco, writing in 2017, drew on these guidelines to create a formula determining qualitative sample sizes. Yocco’s proposed formula is as follows: P = (scope x characteristics) / (expertise ± resources)
The variables in Yocco’s formula are:
- Scope—“what you are trying to accomplish”, which can be a value from 1 to infinity
- Characteristics—the number of user types, multiplied by 3 participants per type
- Expertise—years of researcher experience, starting from 1 and +0.10 every 5 years
- Resources—budget and time constraints, which is ”a number from N (i.e. the desired sample size) - 1 or more to + 1 or more”
I liked the principle behind this approach and am indebted to Yocco for providing the starting point for our own, below.
Our formula for qualitative sampling
Participants = No-show rate x ((scope x diversity x method)/expertise)
You’ll notice that our formula incorporates method as a variable (because when it comes to study sizes, usability testing ≠ interviews ≠ diary studies), as well as no-show rate. Unlike Yocco’s formula, ours does not attempt to quantify “resources.”
We’ll go over each element of our formula in turn. But first, in order to understand these variables (inputs) and the resulting recommendations (outputs), you’ll need to be familiar with the concept of saturation in qualitative sampling, as well as the factors that impact it.
Not sure which research method is best? Our UXR Method Selection Tool can help
Saturation in qualitative research
The concept of saturation has its roots in grounded theory but is now widely used among qualitative researchers “as a criterion for discontinuing data collection and/or analysis” (Saunders et al., 2018).
Adapting traditional qualitative methods to UX often involves cutting through quite a bit of noise—and the topic of saturation is no different. There are many differing definitions of saturation, as well as conflicting approaches to determining when it’s been reached (see O’Reilly & Parker (2013), Sebele-Mpofu (2020), and Saunders, et al. (2018) for further discussion).
In many ways, these differences are—quite literally—academic. When we set aside semantic differences and arguments about exact thresholds or means of calculation, we find that most folks mostly agree with the following definition offered by Maria Rosala of Nielsen Norman Group (2021):
“Saturation in a qualitative study is a point where themes emerging from the research are fleshed out enough such that conducting more interviews won’t provide new insights that would alter those themes.”
The concept of saturation can be further broken down into forms of saturation.

In their frequently cited study on interview saturation, Hennick, et al. (2017) define two forms of saturation, which I have adopted for our approach.
- Code saturation—“the point when no additional issues are identified and the codebook begins to stabilize.”
- Meaning saturation—“the point when we fully understand issues, and when no further dimensions, nuances, or insights of issues can be found.”
According to Hennick, et al. (2017), code saturation “may indicate when researchers have “heard it all”” whereas meaning saturation occurs when researchers “understand it all.”
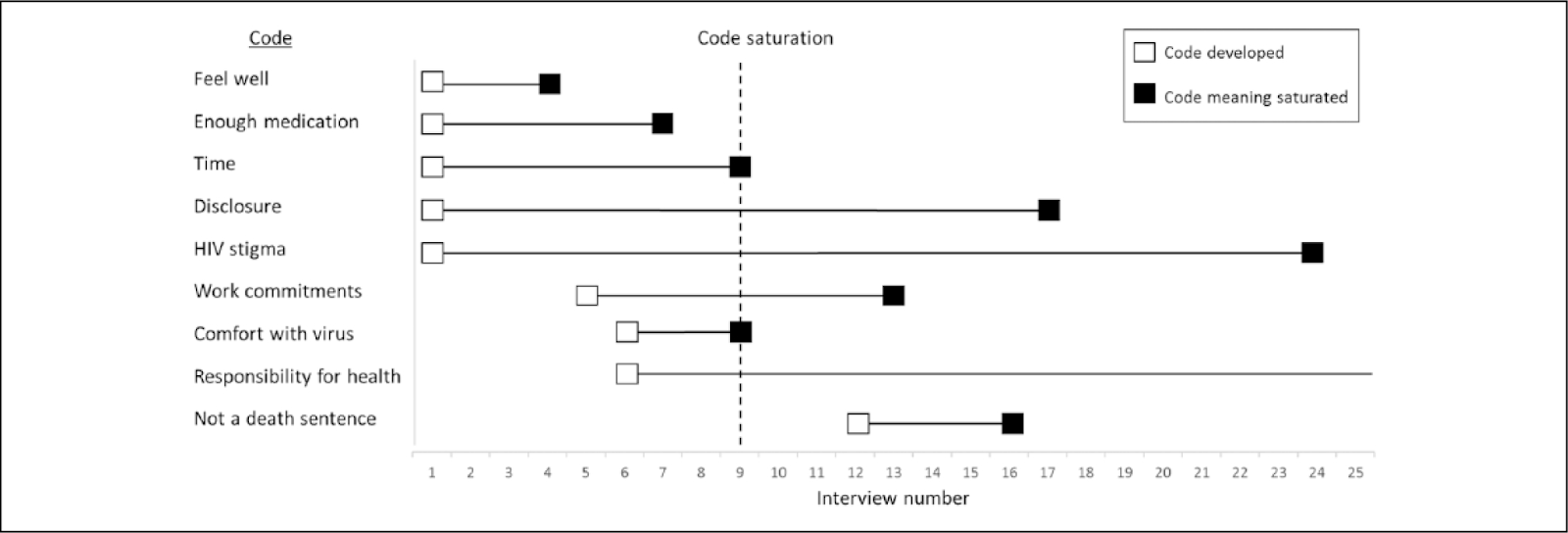
The same study—which looked at 25 interviews in total—concluded that code saturation was reached at 9 interviews (“whereby the range of thematic issues was identified”), but that 16-24 interviews were required to reach meaning saturation where they “developed a richly textured understanding of issues.”
Another study (Hennick et al., 2019), which looked at focus groups, found that code saturation was reached at 4 groups, but that meaning saturation for each code was not reached until around group 5.
In other words, it takes longer to reach meaning saturation than code saturation.
Now, sometimes code saturation may be sufficient (e.g. when you’re planning to follow up on results with further research). But for most decisions, stakeholders want to understand a problem before taking action—in other words, they’re seeking meaning.
So, in order to facilitate efficient, decision-driven research, the sample size recommendations in our calculator are based on meaning saturation for different study types wherever possible.
📕 Note: Saturation is widely used in qualitative research—but it is by no means universally accepted. Please see Appendix I for some of the criticisms of saturation in qualitative research.
Factors that influence the sample size in qualitative research
The number of participants you need to achieve saturation depends on many different factors, some more important than others. (See: Mwita (2022), Hennick et al. (2019), Aldiabat & Le Navenec (2018), and Bonde (2013).)
Five of these factors are baked into our formula:
- The method you use
- Your research scope and study goals
- The diversity of your sample population
- Expertise of researchers involved
- Typical no-show rate for qualitative studies
Two other factors—resources and stakeholder requirements—are not factored into our calculator but will influence how you use the recommendations we give you.
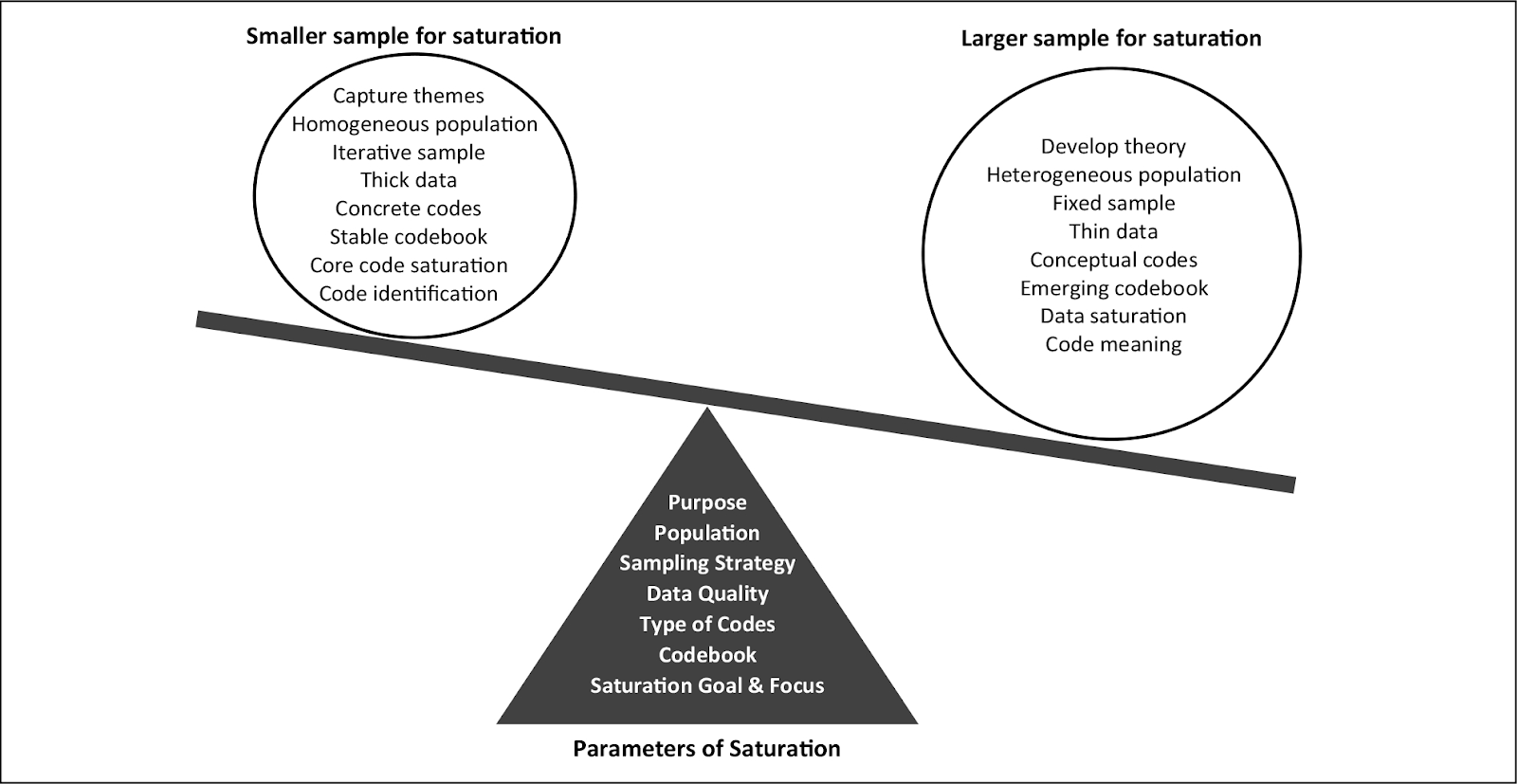
We’ll discuss methods last, so let’s start with scope…
Research scope and study goals
There are many ways to think about and define research scope.
For instance, Rolf Molich (2010) offers an interesting framework—based on comparative usability evaluation studies and personal research experience—which includes sample size guidelines for “political” research which seeks to demonstrate to stakeholders that problems exist and can be identified.

Citing a research colleague at Maze, Ray Slater Berry (2023) discusses scope in terms of tactical vs. strategic research. Maze accordingly offers different study size recommendations for a tactical study (”one you conduct to make quick fixes and immediate improvements”) vs a strategic one that “aims to shape a product’s long-term direction.”

Other sources (e.g. Spillers, 2019) discuss scope and goals in terms of summative vs. formative research, with the latter requiring fewer participants. Brittany Schiessel (2023) of consulting firm Blink UX adds an additional type of research (foundational) to this framework.
- Foundational (10-12 Ps) – to gain a deeper understanding of your topic or area of interest
- Formative (6-8 Ps) — to identify issues and considerations early on to inform the design before starting development
- Summative (15+ Ps) — to measure the user experience
The principles behind these last two frameworks (from Maze and Blink UX) roughly align with the findings of Hennick et al. (2017), namely that code saturation may be sufficient in a study “aiming to outline broad thematic issues or to develop items for a survey,” (aka formative or tactical research) but that “to understand or explain complex phenomena or develop theory” (aka foundational or strategic research) more interviews may be necessary to reach meaning saturation.
Together, Slater Berry (2023), Schiessel (2023), Hennick et al. (2017), and Yocco (2017) informed the way in which I thought about and defined research scope in this context.
In the Qualitative Sample Size Calculator:
In our formula, scope is a value, which varies depending on the characteristics of your study:
- Narrow — Research about an existing product or very specific problem (fewest unknowns, tactical, formative). Scope = 1
- Focused — Creating a new product or exploring a defined problem (some unknowns, tactical or strategic, formative). Scope = 1.25
- Broad — Discovering new problems/insights (many unknowns, strategic, foundational). Scope = 1.5
We tried to make it easy for you to identify your study type among our list of options, regardless of which framework you usually use to talk about your research goals.
Population diversity
Diversity in this context is a neutral term and does not necessarily mean you have not done your due diligence to ensure that your research is inclusive. Rather, diversity here is a measure of how wide a net your study casts; it is inversely related to how niche your screening criteria is—the more screening criteria you use, the less diverse your population is likely to be.
For this reason, B2B studies are likely to be more homogenous than general consumer research studies, by which we mean participants in B2B research tend to be more similar to each other in terms of job titles, education, software habits, etc.
As a rule, the more diverse your population is, the more participants you will need to reach saturation (Hennick & Kaiser, 2022).
Earlier attempts to create a formula for calculating qualitative study size (Yocco (2017), Blink UX (n.d.)) use whole numbers for this variable—the idea being that you should multiply other factors by the number of user types/personas in your population.
But I wasn’t sure that researchers would always be able to estimate the number of personas in their sample, especially when it comes to early discovery research with all its unknowns.
So instead of asking you how many user types are in your population, we’ve asked you to indicate how diverse you believe your population is on a scale of “very similar” to “very diverse.”
In the Qualitative Sample Size Calculator:
In our formula diversity is represented by a value:
- Very similar: I am using multiple criteria to recruit a very specific group of people with shared traits and/or habits (e.g “UK-based software engineers with 5-10 years of experience who have used AWS”) Diversity = 1
- Somewhat similar: I am recruiting a specific group of people (e.g. “Software engineers with 5-10 years of experience”) Diversity = 1.3
- Somewhat different: I am recruiting somewhat varied participants or a couple of different groups. There are some restrictions but a good number of people could qualify (e.g. “Women who have shopped online 2+ times in the last 12 months.”) Diversity = 1.5
- Very different: I am recruiting a very diverse set of participants or a few different groups. Qualifying criteria is broader and many people could qualify. (e.g. “People who have shopped online in the last 12 months.”) Diversity = 1.7
For the jump from “very similar” to “very similar” the value increases by +30%. For every degree of diversity after that, it increases by +15%. This is based on an assumption that there will be some similarities between groups in your population, and that the total amount of overlap will increase as your sample size does.
As Jakob Nielsen (2000) notes, when “testing multiple groups of disparate users, you don't need to include as many members of each group as you would in a single test of a single group of users” because there will likely be significant overlap between segments.
In other words, you don’t need to recruit 2, 3, 4, 5, 6 times as many people just because you have that many personas in your sample.
📕 Note: I dwelled on these values a lot, and made several attempts to ground them in existing research on saturation in heterogeneous populations vs. homogenous ones. This was challenging, and I had to adjust the approach to this variable several times. For a discussion of my rationale, please see Appendix II.
Researcher expertise
In their discussion of data saturation in Grounded Theory, Aldiabat and Le Navenec (2018) identified salient factors that facilitate saturation. Four of those six factors are related to researcher expertise.
Onwuegbuzie, et al. (2010) and Bonde (2013) also found researcher experience to be an important factor, with the latter concluding that:
“The more expertise the researcher has, and the more familiar they are with the phenomena under investigation, the more effectively they can perform as a research instrument.”
In other words, skilled researchers can uncover insights with fewer participants.
In the Qualitative Sample Size Calculator:
In our formula, expertise is a value between 1 - 1.3, depending on your answer to the question: “How many years of experience (on average) do the researchers involved in this project have with this type of research?”
- 0-4 years. Expertise = 1
- 5-9 years. Expertise = 1.1
- 10-14 years. Expertise = 1.15
- 15-19 years. Expertise = 1.2
- 20-24 years. Expertise = 1.25
- 25+ years. Expertise = 1.3
In his formula, Yocco (2017) suggests that this variable should start at 1 for beginner researchers and increase at a rate of 0.10 for every 5 years of experience. For our own calculator, we found that increasing the expertise denominator at this rate produced too dramatic an effect
We still increased this value by 0.10 for that first jump from 0-4 to 5-9 years of experience, but reduced the step up to 0.05 for every 5-year interval after that.
No-show rate
No-shows are participants who accept a study invite but never show up to the session.
In a survey of 201 usability professionals, the folks at Nielsen Norman Group found that people reported an average no-show rate of 11% (Nielsen, 2003). Meanwhile, Jeff Sauro (2018) suggests that the typical range is between 10 to 20%.
That means that for every 10 participants you need, you should actually recruit 11 or 12.
(For what it’s worth, User Interviews’ no-show rate is less than 8%, according to a 2023 analysis of our participant panel.) (See: Burnam, 2023)
We stuck with a rate of 10%—since it’s a tidy number and not everyone recruits with User Interviews—and baked this buffer into our calculator as a fixed value (0.10).
👉 User Interviews is the fastest way to recruit participants. Source from our panel of 4.1 million to reach nearly any target audience.
Qualitative sample sizes by method
The method multiplier depends, naturally, on which method you choose. The value represents the baseline number of participants recommended for achieving saturation in a homogeneous study.
To arrive at these numbers, I combed through dozens of peer-reviewed articles, empirical studies, and advice from industry professionals and made note of their recommended sample sizes and the rationale behind them. I then looked for consensus (where possible) and/or data-backed recommendations relevant to UX research.
In general, I chose a baseline on the lower end of recommended sample size ranges, since the method value is multiplied by the other variables in our formula.
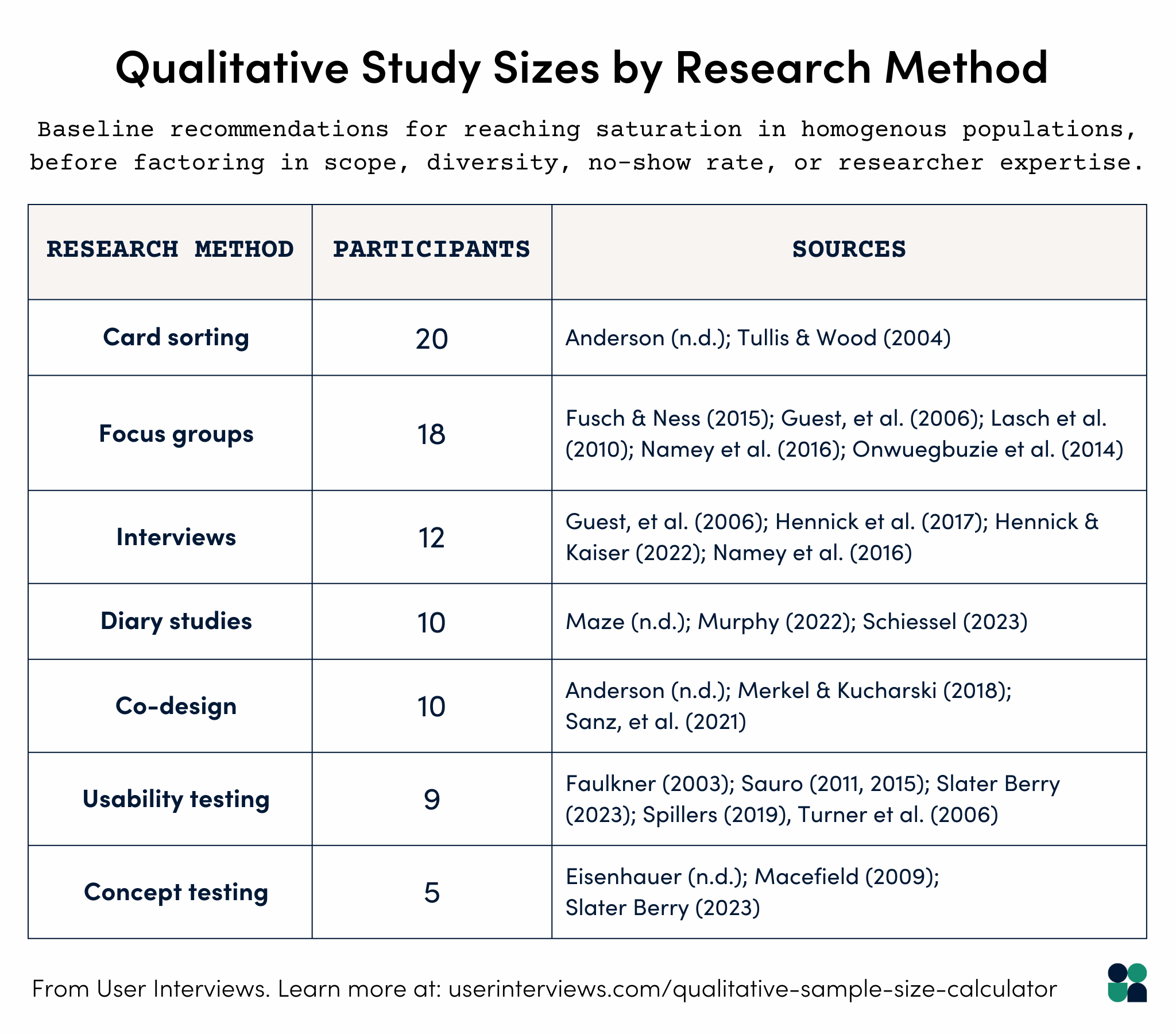
📕 Please see Appendix III for a full discussion of the sources, table with additional works cited, and the rationale behind our recommendation.
💬 Interviews (baseline sample size = 12)
Interviews (also called in-depth interviews or IDIs) are conversations with a single participant.
In our formula, when method = interviews, the value is 12. This is the baseline number of participants recommended to reach saturation with a homogenous population. This is supported by the findings of Guest, et al. (2006), Namey et al (2016), and Hennick & Kaiser (2022).
Note that once other factors like scope and diversity are applied, you will likely end up with a sample size closer to the 16 threshold advised by Hennick et al. (2017) and Hagaman & Wutich (2016).
👂 Focus groups (baseline sample size = 18)
A focus group is a moderated conversation in which a researcher asks a group of participants a set of questions about a particular topic. These aren’t “group interviews”; the value of this method lies in the discussion between participants.
You should think about each focus group discussion as a single data collection event, just as a 1:1 interview is a single data collection event (Namey, et al., 2016). In other words, a focus group with 6 participants does not equal 6 interviews—it equals one discussion.
To work out the total sample size needed for a focus group study, you need to consider both the group size and the number of discussions (data collection events). In our formula, the value for focus groups is 18—that’s 3 groups of 6 participants.
This recommendation is based on the work of Fusch & Ness (2015), Onwuegbuzie et al., (2010), Namey et al. (2016), and Guest et. al (2016).
✍️ Diary studies (baseline sample size = 10)
Diary studies are longitudinal studies in which participants keep a log of their thoughts, experiences, and activities over a period of time—usually a few days to several weeks.
The most useful advice regarding diary study sample recruiting that I could find came from the first-hand experience of qualitative researchers at UX companies or firms—such as Murphy (2022) of Indeemo, Slater Berry (2023) of Maze, and Schiessel (2023) of Blink UX.
All three of these sources recommend at least 10 participants for diary studies—a baseline that aligns with the advice of Bernard (2013) who suggests in his Social Research Methods: Qualitative and Quantitative Approaches, 2nd ed. that 10-20 participants are sufficient for studies that seek to explore and understand lived experiences.
Our baseline recommendation for diary study sample size is 10, as advised by these sources.
🎨 Co-design (baseline sample size = 10)
Co-design, or participatory design, is a collaborative approach in which participants (potential users) are active contributors in the design process. Or, to quote Steen, et al. (2011):
“In co-design, diverse experts come together, such as researchers, designers or developers, and (potential) customers and users—who are also experts, that is, “experts of their experiences” [...] to cooperate creatively. “
It was difficult to find peer-reviewed recommendations for co-design study sizes and the number of participants involved in the case studies I encountered varied widely—from as few as 3 to over 100 participants. (Study sizes of 6-20 participants were most common).
Our sample size recommendation is based on my own analysis of 37 co-design studies involving 3-25 participants. Among these examples, the median number of participants per co-design study was 13, with 10 being the most common study size.
This matches up with the advice of Nikki Anderson who states that 10 to 12 participants (per user segment) is appropriate for co-design studies in a UX research context.
🃏 Card sorting (baseline sample size = 20 participants)
Card sorting involves having participants group together cards (topics) in a way that makes sense to them—either by creating groups themselves (an open card sort) or sorting cards into predefined categories (closed card sort).
There seems to be a degree of consensus around the required number of participants for a card sort—all the sources I consulted recommend between 10-30 participants for a typical study.
I grounded our baseline value on the work of Tullis & Wood (2004), who conducted a study with 168 participants and then analyzed similarity matrices and tree structures from various sample sizes to assess minimum study size. Based on their research, the authors recommend 20-30 participants for a card sort.
We set our baseline value for this method at 20, accordingly.
💻 Usability testing (baseline sample size = 9 participants)
Usability testing involves testing a product with users to evaluate it’s, well, usability. It’s a vital step in the product development process, as it helps teams identify problems and opportunities for improvement.
You’ve almost certainly heard of the “5-user rule,” which was popularized by Jakob Nielsen in his 2000 article titled “Why You Only Need to Test with 5 Users.”
This rule has been frequently challenged by researchers (Sauro, 2010) and Nielsen himself has since clarified that, in fact, you will may need to run 3 tests with 5 users—to uncover all the usability problems in a design—in other words, the actual number of users you need may be closer to 15.
Nonetheless, the 5 user rule is well known and remains a popular fallback.
So why, in our formula, did I use a baseline sample size of 9 participants?
This value is based on the binomial approach recommended by Jeff Sauro (2011). According to Sauro’s math, you would need to test with 9 users for an 85% chance of observing behaviors or problems that occur with 20% of users.
A higher sample size is also supported by the work of Faulkner (2003), who found that—in a study involving 60 user tests—randomly selected sets of 10 participants identified at least 82% of problems (95% on average). Some randomly selected sets of 5 participants, on the other hand, found as little as 55% of problems (86% on average).
I feel more comfortable advising for a slightly higher sample size with a greater minimum chance of identifying problems (which is, you know, the point), thus our baseline value of 9.
💡 Concept testing (baseline sample size = 5 participants)
Concept testing is typically conducted during the early stages of a project to gauge user reactions to ideas or simple prototypes. This method helps you assess whether you’re on the right track and is often done before committing to further design and development.
For qualitative concept testing, the sources I could find were all fairly consistent—5-10 participants seems sufficient for this method.
Not quite sure of the best method to use? Check out our free UX Research Method Selection Tool.
Putting it all together
Now that we have a good understanding of each input, let’s take a look at our formula in action with three scenarios.
Participants = No-show rate x ((scope x diversity x method)/expertise).
Scenario 1: Discovery interviews
You want to do some discovery interviews as part of developing a new product—let’s say it’s a tool for Sales Ops. Your target users are Sales Ops specialists, but you also want to speak to other folks who are involved with sales operations as part of their work. In other words, your participants likely have somewhat similar use cases and needs. You have 10 years of research experience and are conducting these interviews alongside a PM with 4 years of experience doing customer interviews—you plan to divide this work equally.
- Scope = Broad = 1.5
- Diversity = Somewhat similar = 1.3
- Method = Interviews = 12
- Expertise = 5-9 years [on average] = 1.1
Remember no-show rate is fixed at 1.10. Therefore:
Participants = 1.1 x ((1.5 x 1.3 x 12)/1.1) = 23.4
People don’t come in fractions, so we round up that sample size to 24.
Scenario 2: Concept testing
You are a UX designer working on a travel product for the UK market. After doing some discovery research with your target audience (let’s say they’re high-income adults with a strong interest in luxury, eco/culturally conscious travel) you have a clear concept and a low-fi prototype. Now, you want to find out if you’re headed in the right direction—do these ideas resonate? You have 3 years of experience doing this kind of research.
- Scope = Focused = 1.25
- Diversity = Very similar = 1
- Method = Concept testing = 5
- Expertise = 0-4 years = 1
So in this case, you’d need to recruit 7 participants.
Participants = 1.1 x (( 1.25 x 1 x 5)/1) = 6.9
Scenario 3: Card sorting
Let’s say you are a user researcher with 12 years of experience. Your company is working on a major product redesign for your established edtech software, which is aimed at helping US high school students (aged 14-18) get excited about History subjects.
A fluid navigation is important to the user experience—you want to help children discover topics and find connections between them. Before your design team can get to work on the IA (information architecture), you need to understand students’ mental models and how they categorize information. You plan to conduct a card sort study to inform the redesign.
- Scope = Narrow = 1
- Diversity = Somewhat similar = 1.3
- Method = Card sorting = 20
- Expertise = 10-14 years = 1.15
Participants = 1.1 x ((1 x 1.3 x 20)/1.15) = 24.9
Rounding up, that’s a sample size of 25 for your card sorting study.
🧮 Try our calculator to get a personalized sample size recommendation for your next qualitative study.
How to apply these sample sizes to your study
Here’s the thing about qualitative research—when you ask a pro what the right thing to do is, the answer is always going to be “it depends.”
There are contextual variables not represented in this calculator. Your budget, timeline, bandwidth, and the importance of the project should all be used to interpret and apply the calculator's results.
Questions to ask yourself include:
- What do your stakeholders value? Speed or rigor?
- Do they care a lot about statistical significance?
- Will the results of your research be used to make critical, irreversible decisions?
- Or are you mostly looking for a gut check?
- If you recruit more participants, do you have the time to conduct the sessions properly and analyze the results carefully?
- Do you have the people power to juggle more interviews?
- Do you have the budget?
Our calculator gives you a single number rather than a range—in large part because so few resources do, and we wanted to provide you with guidance that you may not be able to find elsewhere.
However, when it comes to setting stakeholder expectations and requesting budget, we advise that you do give a range with some buffer baked in. If you think you’re going to need to talk to 16 people based on your calculator results, set expectations for 16-20 (or more). Start by recruiting for 16, analyze as you go, and leave some time/budget buffer for the other 4 folks if it turns out you need them.
Limitations of our formula
I’m pretty excited about this calculator—I believe it fulfills a genuine need among User Researchers and people who do research. And I hope I’ve convinced you that this is a tool you can feel good about using, with truly data-backed recommendations you can be confident in.
That said, this calculator does have its limitations. All things do.
Let’s address three big ones:
1. It’s not intended for clinical research
Our calculator is not intended for use in clinical research which may have life-or-death consequences. Please refer to the recommendations of your university, hospital, or research facility for advice about studies where saturation and confidence levels above 99% are sought.
2. It’s aimed at UX and product folks
The recommendations we’ve offered are intended to be used by folks working in user research, UX design, product development, and similar environments where the primary goal of research is to facilitate immediate or near-term decision making.
That typically means shorter timelines and less rigor—in quantitative terms, I expect these folks to be aiming for 70-85% confidence levels rather than the 95+% typically advised for academic and peer-reviewed research (Anderson, n.d; Sauro, 2015).
If you’re doing qualitative research in a rigorous environment or if your stakeholders demand statistical significance, these recommendations may be too low to satisfy those requirements.
3. It is not optimized for mixed methods
Finally, this calculator is not currently optimized for mixed methods research. That’s not to say mixed methods researchers can’t use it—you totally can!—but you’ll need to work out how your total sample size will be affected once you layer in other methods.
How to find the right participants for your study
Now that you have a sample size you feel good about, the next obvious step is finding the right participants—however many that may be.
And wouldn’t ya know it… User Interviews just happens to be the fastest way to recruit participants.
But we’re not just fast. We offer advanced targeting for attributes like industry, job title, technical characteristics, and demographics as well as custom screener surveys—so you can find exactly the folks you’re looking for among our panel of 4.1 million (and counting).
We also help you automate the not-fun parts of research. Think: Scheduling, messaging participants, and incentives payouts.
Recruit your next study with User Interviews (it’s free to try—you only pay for completed sessions).
✨ TRY USER INTERVIEWS FREE ✨
Appendices
Appendix I: Criticisms of saturation
Any discussion of saturation should address the fact that—while it is widely used—saturation as a measure of “done-ness” has its detractors. There are researchers who challenge the use of saturation in qualitative sampling (e.g. O’Reilly & Parker, 2013) and those who warn of relying too firmly on pre-established sample sizes for qualitative insights (e.g. Sim, et al., 2018) who cautions:
“Studies that rely on statistical formulae […] or that otherwise arrive at predicted numbers of interviews at which saturation will occur […] make a naïve realist assumption – i.e. that themes ‘pre-exist’ in participants’ accounts, independently of the analyst, and are there to be discovered.”
Jacqueline Low (2019), meanwhile, argues that the idea of saturation as a point at which “no new information” emerges is “a logical fallacy, as there are always new theoretic insights as long as data continue to be collected.”
These criticisms are valid and it would be foolish of us to think that any research—or any amount of research—will ever be able to uncover all the insights or themes or data that exists. (If that were true, we’d probably have finished researching things by now.)
But it is important to understand that qualitative ≠ quantitative. There are no bullet-proof statistical models that can tell you exactly how many people you need to interview to be X% confident that you understand a complex problem.
Nevertheless, researchers do need to be able to estimate sample sizes ahead of time (for the purposes of budgeting, planning timelines, getting stakeholder buy-in, etc.).
Get yourself and your stakeholders comfortable with the idea that—in some cases—you may need to recruit a few more participants as you go (and in others, you may find you’ve reached saturation before your final interview).
Once you’ve accepted that, it is possible to make reasonably confident predictions about how many people you need to recruit ahead of time in order to be reasonably confident that you’ve uncovered enough insights to take action.
Our calculator is designed to do exactly this—help you work out how many participants you need to feel confident in the conclusions of your research.
Appendix II: The challenge of quantifying diversity
I tried really, really hard to ground the diversity values in previous research about the influence of population diversity on saturation. I had hoped to uncover data that would allow me to extrapolate a ratio—something concrete about how the sample size needed for saturation scales with each additional persona or segment.
This turned out to be quite challenging. Sources like Marshall et al. (2013), Hagaman & Wutich (2016), and Hennick & Kaiser (2019) discuss the impact of heterogeneity on saturation, but fail to explain what that heterogeneity looks like in terms of user groups, personas, segments, and so on.
Hagaman and Wutich (2016), for instance, found that in homogenous populations, 16 interviews were sufficient to identify a theme; but in heterogenous populations, 20-40 interviews were needed to identify metathemes that cut across the study sample.
In other words, you’d need 25-88% more participants (56% on average) to reach saturation in a diverse population than in a homogenous ones. But, how does that number scale as diversity increases? I couldn’t work it out.
Then we have a 2013 study from Marshall et al., which examined 83 qualitative studies and found that on average “single case” studies involved 23 interviews, while “multiple case” studies involved 40 interviews—or 74% more.

Marketing research firm IntoTheMinds offers an interview study size calculator with recommendations partially based on Marshall et al (2013). For heterogeneous populations (“Do/will customers use the product/service to meet the same type of need?” = “No”), they recommend 55-74% more participants than homogenous populations—or 65% on average.
But this still fails to address degrees of diversity. Moreover, Marshall et al. deals with the number of interviews conducted—not necessarily the number needed to reach saturation. Indeed, the authors cautioned that “little or no rigor for justifying sample size was shown for virtually all of the [interview] studies in this dataset.”
So, who’s to say that those ratios are optimal?
Consulting firm BlinkUX offers a usability testing study size calculator, in which they increase the recommended study size according to the number of user groups. They start at a baseline recommendation of 10 and increase by 6 for each additional group.
This was the only source that I found which accounted for the number of user groups (or degrees of heterogeneity), but I was unclear on the basis for these recommendations or the logic behind the +6 user step up. Nonetheless, I ultimately adapted BlinkUX’s approach for our own calculator since it got us closest to the spirit of what I was trying to achieve.
I ended up working out the values for this variable through trial and error until we arrived at inputs that—when applied to the formula and combined with other multipliers—returned study sizes within the recommended ranges for each method.
Appendix III: Methods - Literature review and discussion
In-depth interview sample size – data & sources
Our baseline interview sample size—12—reflects the number of participants needed to reach saturation with a homogenous population, as supported by the findings of Guest, et al. (2006), Namey et al (2016), and Hennick & Kaiser (2022).
The earliest of these sources (Guest, et al., 2006) involved a systematic documentation of saturation over the course of a thematic analysis of 60 interviews. In this study, metathemes emerged as early as the sixth interview, while by the twelfth interview, 88% of emergent themes and 97% of important themes were developed.
In Namey et al. (2016), the authors conducted an inductive thematic analysis of 40 in-depth interviews and 40 focus group discussions. They found that the median number of interviews required to reach saturation was between 8 and 16 (for 80% and 90% saturation, respectively).
Finally, Hennick and Kaiser—in a much-cited 2022 review of 23 studies which used empirical analysis to assess saturation—found that saturation was reached between 9 and 17 interviews, with a median of 12-13.
Note that in an earlier study, Hennick, Kaiser, & Marconi (2017) examined 25 interviews to assess saturation and found that while code saturation occurred between 6 and 9 interviews (84 and 91% saturation, respectively), 16 to 24 interviews were required to reach meaning saturation. And Hagaman & Wutich (2016) found that 16 or fewer interviews were enough to identify common themes in homogenous groups, based on a cross-cultural study with 132 respondents.

Focus group sample size – data & sources
Focus group study size includes both group size and the number of discussions. In our formula, the value for focus groups is 18—that’s 3 groups of 6 participants.
Number of participants per group = 6
This number is based on two sources—Fusch & Ness (2015) and Onwuegbuzie et al., (2010), the latter of whom cites Krueger (1994) and Morgan (1997)—which recommend a group size of 6 to 12 participants.
Getting group size right is important. As Graham & Bryan (2022) note, “focus groups that are too small may have domination problems and larger focus groups are difficult for novice researchers to moderate.”
We felt that 6 participants was a comfortable baseline—neither too small, nor too large to manage.

Number of focus group discussions = 3
For this recommendation, I relied on the findings of Emily Namey, Greg Guest, and their colleagues in Namey et al. (2016) and Guest et. al (2016).
The first of these sources—Namey et al. (2016)—involved inductive thematic analysis of 40 focus group discussions (and 40 interviews, as discussed above). The authors found that the median number of focus group discussions required to reach saturation was between 3 and 5 (for 80% and 90% saturation, respectively).
Guest et al. (2016) relies on a thematic analysis of the same 40 focus group discussions. In this case, the authors found that 80% of all themes were discoverable within 2 to 3 focus groups, with 90% discoverable within 3 to 6 groups.

Diary study sample size – data & sources
Finding peer-reviewed recommendations specific to this method proved tricky. An unsystematic look through individual studies which employ diary studies shows that sample sizes vary widely—the studies I found involved anywhere from 5 to 658 participants (Bagge & Ekdahl (2020), Conner & Silvia (2015), Demerouti (2015), Horwood, et al. (2022), Menheere, et al. (2020), Qin, et al. (2019), Tuthill, et al. (2020), van Hooff & Geurts (2015), van Woerkom, et al. (2015), and Zhang, et al. (2022))
Even among closely related studies the range can be dramatic—a review of studies in the field of organizational research by Ohly, et al. (2010) included diary studies with sample sizes from 7 to 420 participants (median = 99, average = 108).
Not exactly helpful guidelines…
As mentioned above, the most salient recommendations for diary study sample sizes came from first-hand researcher experience—such as that of Murphy (2022) of Indeemo, Slater Berry (2023) of Maze, and Schiessel (2023) of Blink UX.
These sources all recommend at least 10 participants for diary studies, which is supported by Bernard (2013) who advises that 10-20 participants are sufficient for studies that seek to explore and understand lived experiences.
Our baseline recommendation for diary study sample size is 10, in agreement with those sources.
Note that, given their longevity, diary studies can have a higher-than-normal drop-off rate. You may want to adjust the no-show rate in our formula to 1.2 or more to account for this factor.

Co-design (participatory design) sample size – data & sources
As with diary studies, finding peer-reviewed recommendations for co-design study sizes proved challenging and I looked to individual case studies to get a sense of typical sample sizes for this method.
Participatory design has traditionally been more common in health and public sectors, and many of the sources I could find came from those fields, rather than UX research.
The study sizes I encountered vary widely—from as few as one to over 100 participants—but study sizes of 6-20 participants were most common.
This observation is backed by Tuhkala (2010), whose systematic review of 72 participatory design studies involving teachers found that among those studies with a defined number of participants (n=50), half involved just 1-5 participants, while studies with 6-20 participants accounted for a further 36% of those in Tuhkala’s review.
I analyzed another 37 co-design studies with 3-25 participants—many of which come from the field of health tech, as I relied on Merkel & Kucharski (2018) and Ferri Sanz et al. (2021) for source material.
Among these studies I analyzed, the median and average number of participants per co-design study was 13, while the most common study size was 10.
These numbers are supported by Nikki Anderson’s recommendation—she draws on her first-hand experience when she suggests that 10 to 12 participants (per user segment) is appropriate for co-design studies in a UX research context.
Note: Saturation does not seem to be a consideration for co-design studies—likely because this concept does not neatly apply to a method that is often centered on generating ideas and solutions rather than uncovering them through systematic inquiry. It was not possible, therefore, to consider saturation in our recommendations.
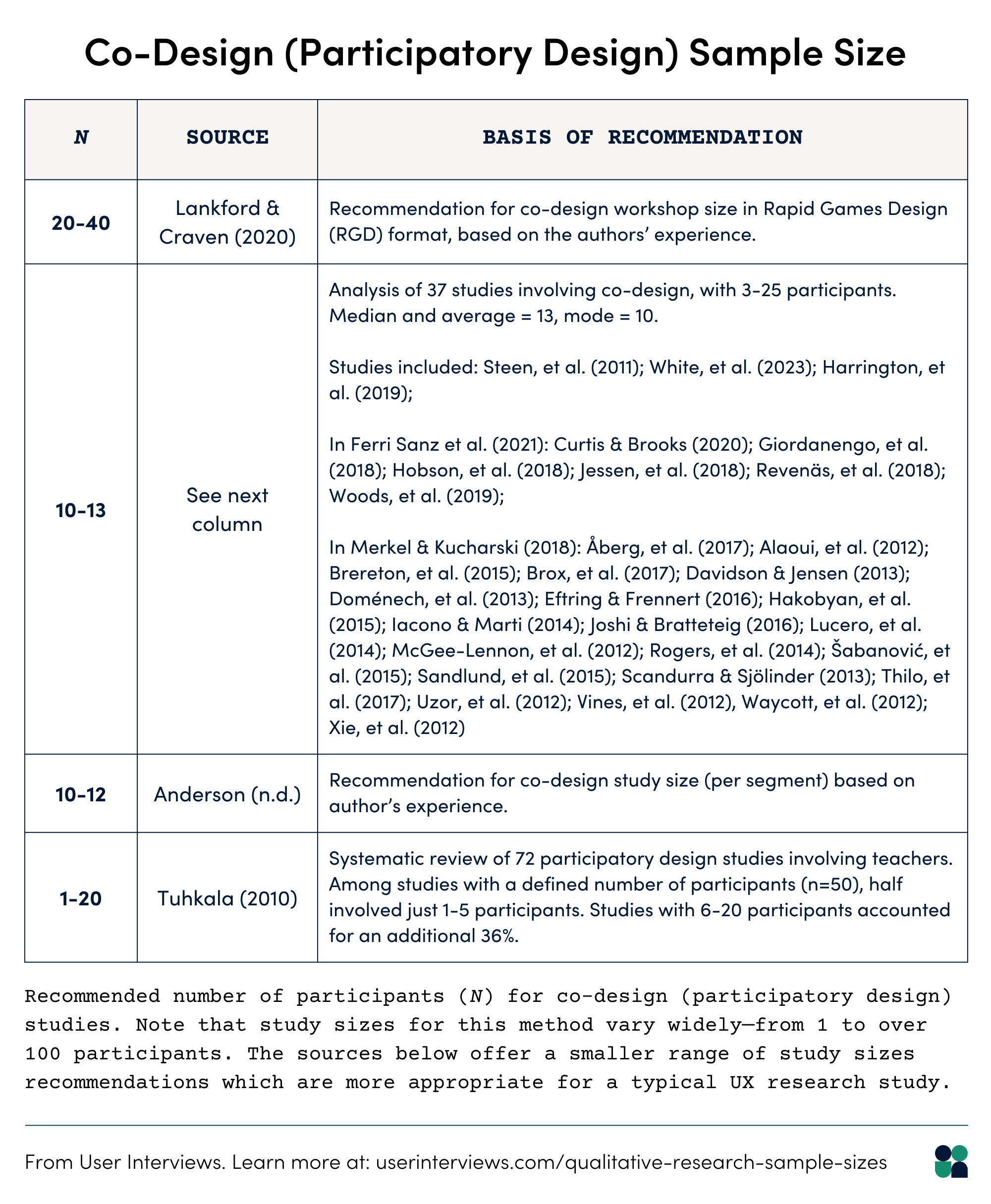
Card sorting study sample size – data & sources
All our sources recommend between 10-30 participants for a typical card sorting study.
On the lower end, Lanz et al. (2019) recommends 10-15 participants for “optimal estimation of the similarity matrix” based on the authors’ analysis of existing card sorting and pairwise analysis studies.
Jakob Nielsen (2019) and Spencer & Warfel (2004) recommend 15 participants, with the latter suggesting that this sample should be split into 3 groups of 5 participants. Nielsen bases his recommendation on the sample size needed to achieve a correlation of 0.90 according to Tullis & Wood (2004).
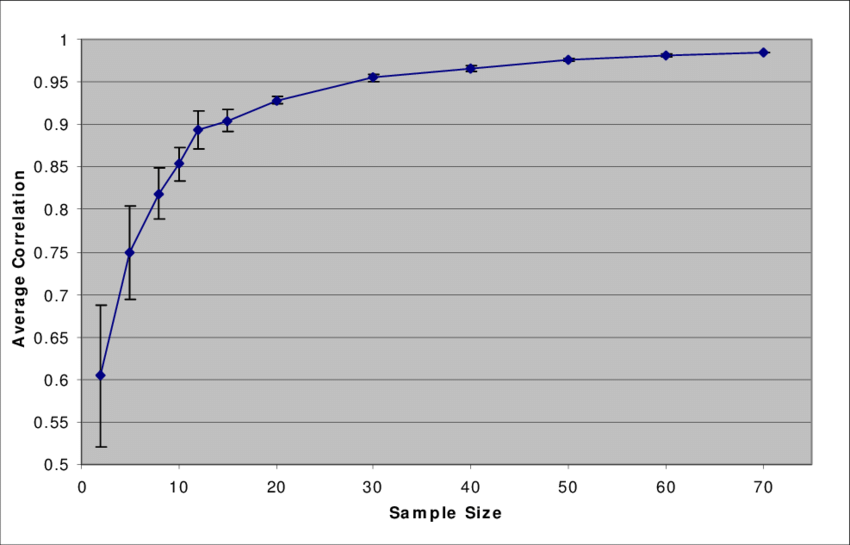
Of all these sources, Tullis & Wood (2004) offers the most robust data to back up their recommendation. Using a set of 168 participants, the authors analyzed similarity matrices and tree structures from various sample sizes to assess minimum study size. They concluded that “reasonable structures are obtained from 20-30 participants”—or when the average correlation between participants was 93-95%.
In our formula, we use a value of 20 for card sorting. If you feel comfortable with lower correlation rates, feel free to replace this with 10 or 15, as advised by Nielsen and others.

Usability testing sample size – data & sources
Two early studies— Virzi (1992) and Nielsen & Landauer (1993)—suggested that with 5 participants, you could uncover 80-85% of the usability problems that exist within a product.
Jakob Nielsen further popularized this idea with an infamous 2000 article “Why You Only Need to Test with 5 Users.” In that article (which you’ve probably heard of, if not read), Nielsen asserts the typical usability issue has a problem occurrence of 31%—in other words, you can uncover 31% of all usability issues by testing with just one user—and that with 5 users, you can observe 85% of such problems.
Nielsen has since clarified that, in fact, you will likely need to test with 15 users to uncover all the usability problems in a design—but he maintains that it is better to run 3 tests with 5 users each, fixing the problems uncovered in each test before testing again with another group of five.
What has become known as the “5-user rule” has been challenged by numerous researchers in the years since it was published (Sauro, 2010).
For example, in a study involving 49 user tests, Spool & Schroeder (2001) found that the first 5 users only uncovered 35% of the usability problems present and that considerably more users would be needed to uncover 85% of problems as Nielsen argues.
Another study by Faulkner (2003), involving 60 user tests, found that randomly selected sets of 5 participants identified between 55-99% of problems—86% on average. With a sample size of 10 users, the minimum percentage of problems uncovered rose to 82% (95% on average), while samples of 20 users identified at least 95% of issues (98% on average).
Jeff Sauro (2011) recommends a binomial approach that accounts for both problem/insight occurrence and chance of observing that problem or behavior. In the table below we see that for an 85% chance of observing behaviors or problems that occur with 20% of users, you’d want to recruit 9 participants.
We have based our baseline sample size (9) on Sauro’s approach.
Note: that we’re talking about qualitative usability testing here. Quantitative usability testing, usability benchmarking, and comparative studies will require more participants.
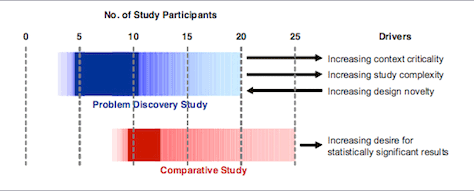
You may want to check out this calculator from SurveyMonkey for working out statistically significant samples for surveys. If your team needs to uncover a specific percent of total problems for your usability testing, we'd recommend checking out these tools from Jeff Sauro at MeasuringU.

Concept testing sample size – data & sources
Most of the sources I could find around concept testing were concerned with quantitative testing, which can involve over 100 participants. (This is also true for quantitative usability testing.)
For qualitative concept testing, the sources I could find were all fairly consistent—5-10 participants seems sufficient for this method according to the pros.
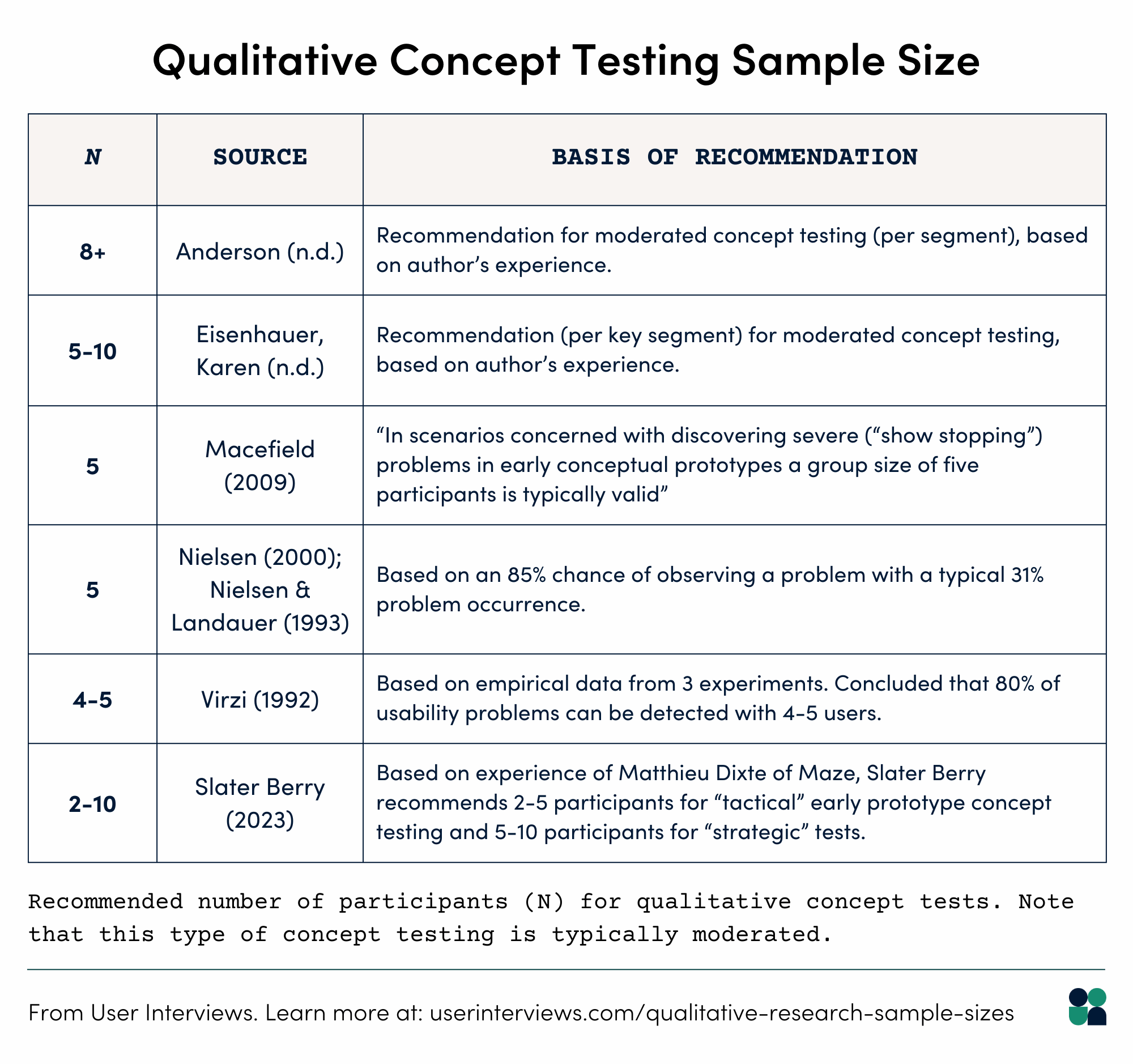
Sources cited
Aldiabat, Khaldoun. M., & Carole-Lynn Le Navenec (2018). “Data Saturation: The Mysterious Step In Grounded Theory Method.” The Qualitative Report, 23(1), 245-261.
Anderson, Nikki (n.d.). “How Many Participants Do You Need? Choosing the ‘Right’ Sample Size.” dscout.
Bagge, Johan, & Kalle Ekdahl (2020). The Predictable Experience of News How User Interface, Context and Content Interplay to Form the User Experience. Master’s Thesis in Industrial Design Engineering, Chalmers University of Technology.
Bernard, H. Russell (2013). Social Research Methods: Qualitative and Quantitative Approaches. SAGE.
Blink UX (n.d.). “Usability Sample Size Calculator.” Blink UX.
Bonde, Donna (2013). Qualitative Market Research: When Enough Is Enough. Research by Design.
Burnam, Lizzy (2023). “Top 5 Takeaways from the 2023 User Interviews Research Panel Report.” User Interviews.
Conner, Tamlin S., & Paul J. Silvia (2015). “Creative days: A daily diary study of emotion, personality, and everyday creativity.” Psychology of Aesthetics, Creativity, and the Arts, 9(4), 463–470.
Demerouti, Evangelia, et al. (2015). “Productive and counterproductive job crafting: A daily diary study.” Journal of Occupational Health Psychology, 20(4), 457–469.
Eisenhauer, Karen (n.d.). “Sample Study Designs: Concept Testing Three Ways.” Dscout.
Faulkner, Laura (2003). “Beyond the Five-User Assumption: Benefits of Increased Sample Sizes in Usability Testing.” Behavior Research Methods, Instruments, & Computers, 35(3), 379–383.
Fusch, Patricia, & Lawrence Ness (2015). “Are We There Yet? Data Saturation in Qualitative Research.” The Qualitative Report, 20(9).
Griffin, Abbie, & John R. Hauser (1993). “The Voice of the Customer.” Marketing Science, 12(1), 1-18.
Graham, Donna, & John Bryan (2022). “How Many Focus Groups Are Enough: Focus Groups for Dissertation Research.” Faculty Focus | Higher Ed Teaching & Learning.
Guest, Greg, et al. (2006). “How Many Interviews Are Enough? An Experiment with Data Saturation and Variability.” Field Methods, 18(1), 59–82.
Guest, Greg, et al. (2016). “How Many Focus Groups Are Enough? Building an Evidence Base for Nonprobability Sample Sizes.” Field Methods, 29(1), 3–22.
Hahsler, Michael & Bernd Simon. (2000). “User-centered Navigation Re-Design for Web-based Information Systems”. In Proceedings of the Americas Conference on Information Systems, H. Michael Chung (Ed.), Long Beach, California, August 2000. pp. 192-198.
Hagaman, Ashley K., & Amber Wutich (2016). “How Many Interviews Are Enough to Identify Metathemes in Multisited and Cross-Cultural Research? Another Perspective on Guest, Bunce, and Johnson’s (2006) Landmark Study.” Field Methods, 29(1), 23–41.
Harrington, Christina N., et al. (2019) “Engaging Low-Income African American Older Adults in Health Discussions through Community-Based Design Workshops.” Proceedings of the 2019 CHI Conference on Human Factors in Computing Systems, May 2019.
Hennink, Monique M., et al. (2017). “Code Saturation versus Meaning Saturation: How Many Interviews Are Enough?” Qualitative Health Research, 27(4), 591–608.
Hennink, Monique, & Bonnie N. Kaiser (2022). “Sample Sizes for Saturation in Qualitative Research: A Systematic Review of Empirical Tests.” Social Science & Medicine, 292(1).
Hennink, Monique M., et al. (2019). “What Influences Saturation? Estimating Sample Sizes in Focus Group Research.” Qualitative Health Research, 29(10), 1483–1496.
Horwood, Christiane, et al. (2022). “It’s not the Destination it’s the Journey: Lessons From a Longitudinal ‘Mixed’ Mixed-Methods Study Among Female Informal Workers in South Africa.” International Journal of Qualitative Methods, 21.
IntoTheMinds (n.d.). “How Many Interviews Should You Conduct for Your Qualitative Research?” IntoTheMinds.
Krueger, Richard A. (1994). Focus groups: A practical guide for applied research. 2nd edition. SAGE.
Krueger, Richard A & Mary Anne Casey. (2000). Focus groups: A practical guide for applied research. 3rdd edition. SAGE.
Lankford, Bruce and Joanne Craven (2020). Managing a ‘rapid games designing’ workshop; constructing a dynamic metaphor to explore complex systems. Rapid Games Design.
Lantz, Ethan, et al. (2019). “Card sorting data collection methodology: how many participants is most efficient?” Journal of Classification, 26(3), 649–658.
Lasch, Kathryn Eilene, et al. (2010) “PRO Development: Rigorous Qualitative Research as the Crucial Foundation.” Quality of Life Research, 19(8), 1087–96.
Low, Jacqueline (2019). “A Pragmatic Definition of the Concept of Theoretical Saturation.” Sociological Focus, 52(2), 131–139.
Macefield, Ritch (2009). “How to Specify the Participant Group Size for Usability Studies: A Practitioner’s Guide.” Journal of Usability Studies.
Macías, José A. & Alma L. Culén (2021). “Enhancing decision-making in user-centered web development: a methodology for card-sorting analysis.” World Wide Web, 24, 2099–2137
Marshall, Bryan, et al. (2013) “Does Sample Size Matter in Qualitative Research?: A Review of Qualitative Interviews in Is Research.” Journal of Computer Information Systems, 54(1), 11–22.
Mark Mason (2010). Sample Size and Saturation in PhD Studies Using Qualitative Interviews. Forum Qualitative Sozialforschung / Forum: Qualitative Social Research, 11(3).
Merkel, Sebastian, & Alexander Kucharski (2018). “Participatory Design in Gerontechnology: A Systematic Literature Review.” The Gerontologist, 59(1), e16–25.
Menheere, Daphne, et al. (2020). “A Diary Study on the Exercise Intention-Behaviour Gap: Implications for the Design of Interactive Products.” Synergy - DRS International Conference 2020, 11-14 August.
Molich, Rolf (2010). “A Commentary of ‘How to Specify the Participant Group Size for Usability Studies: A Practitioner’s Guide’ by R. Macefield - JUX.” Journal of User Experience.
Morgan, David L. (1997). Focus groups as qualitative research. 2nd edition. SAGE.
Murphy, Eugene (2022). “Tips for How to Plan and Conduct a Diary Study Research Project (Guide).” Indeemo.
Mwita, Kelvin (2022). “Factors Influencing Data Saturation in Qualitative Studies.” International Journal of Research in Business and Social Science, 11(4), 414–420.
Namey, Emily, et al. (2016). “Evaluating Bang for the Buck: A Cost-Effectiveness Comparison between Individual Interviews and Focus Groups Based on Thematic Saturation Levels.” American Journal of Evaluation, 37(3), 425–440.
Nielsen, Jakob (2003). “Recruiting Test Participants for Usability Studies.” Nielsen Norman Group.
––– (2000). “Why You Only Need to Test with 5 Users.” Nielsen Norman Group.
— (2019). “Card Sorting: How Many Users to Test.” Nielsen Norman Group.
Nielsen, Jakob, & Thomas K. Landauer (1993). "A mathematical model of the finding of usability problems." Proceedings of ACM INTERCHI'93 Conference (Amsterdam, The Netherlands, 24-29 April 1993), 206-213.
Nyumba, Tobias, et al. (2018). “The Use of Focus Group Discussion Methodology: Insights from Two Decades of Application in Conservation.” Methods in Ecology and Evolution, 9(1), 20–32.
Ohly, Sandra, et al. (2010) “Diary Studies in Organizational Research.” Journal of Personnel Psychology, 9(2), 79–93.
Onwuegbuzie, Anthony, et al. (2010). “Innovative Data Collection Strategies in Qualitative Research.” The Qualitative Report, 15(3).
O’Reilly, Michelle, & Nicola Parker (2013). “‘Unsatisfactory Saturation’: A Critical Exploration of the Notion of Saturated Sample Sizes in Qualitative Research.” Qualitative Research, 13(2), 190–197.
Qin, Xiangang, et al. (2019). “Unraveling the Influence of the Interplay Between Mobile Phones’ and Users’ Awareness on the User Experience (UX) of Using Mobile Phones.” In Human Work Interaction Design. Designing Engaging Automation: Revised Selected Papers of the 5th IFIP WG 13.6 Working Conference. HWID 2018. 69-84.
Rosala, Maria (2021). “How Many Participants for a UX Interview?” Nielsen Norman Group.
Ferri Sanz, Mireia et al. (2021). “Co-Design for People- Centred Care Digital Solutions: A Literature Review.” International Journal of Integrated Care, 21(2):16, 1-17.
Saunders, Benjamin, et al. (2018). “Saturation in Qualitative Research: Exploring Its Conceptualization and Operationalization.” Quality & Quantity, 52(1), 1893–1907.
Sauro, Jeff (2018). “8 Ways to Minimize No Shows in UX Research.” MeasuringU.
— (2010). “A Brief History Of The Magic Number 5 In Usability Testing.” MeasuringU.
––– (2011). “How Many Customers Should You Observe?.” MeasuringU.
Schiessel, Brittany (2023). “How Many Research Participants Do I Need for Sound Study Results?” Blink UX.
Sebele-Mpofu, Favourate Y. (2020). “Saturation Controversy in Qualitative Research: Complexities and Underlying Assumptions. A Literature Review.” Cogent Social Sciences, edited by Sandro Serpa, 6(1).
Slater Berry, Ray (2023). “User Testing: How Many Users Do You Need?” Maze.
Spencer, Donna & Todd Warfel (2004). “Card Sorting: A Definitive Guide.” Boxes and Arrows.
Spillers, Frank (2019). “The 5 User Sample Size Myth: How Many Users Should You Really Test Your UX With?” Experience Dynamics – Medium.
Sim, Julius, et al. (2018). “Can Sample Size in Qualitative Research Be Determined a Priori?” International Journal of Social Research Methodology, 21(5), 619–634.
Six, Janet M., and Ritch Macefield (2016). “How to Determine the Right Number of Participants for Usability Studies.” UX Matters.
Spool, Jared & Will Schroeder (2001). “Testing Web Sites: Five Users Is Nowhere near Enough.” CHI ’01 Extended Abstracts on Human Factors in Computing Systems.
Steen, Marc, et al. (2011). “Benefits of co-design in service design projects.” International Journal of Design, 5(2), 53-60.
Tuhkala, Ari (2021). “A Systematic Literature Review of Participatory Design Studies InvolvingTeachers.” European Journal of Education, 56(1).
Tullis, Thomas, and Larry Wood (2004). “How Many Users Are Enough for a Card-Sorting Study?” Presented at the Annual Meeting of the Usability Professionals Association.
Turner, Carl, et al. (2006). “Determining Usability Test Sample Size.” International Encyclopedia of Ergonomics and Human Factors, 2nd Edition, edited by Waldemar Karwowski. CRC Press, 3076–80.
Tuthill, Emily L., et al. (2020) “Longitudinal Qualitative Methods in Health Behavior and Nursing Research: Assumptions, Design, Analysis and Lessons Learned.” International Journal of Qualitative Methods, 19.
White, P. J., et al. (2023). “Co-Design with Integrated Care Teams: Establishing Information Needs.” International Journal of Integrated Care, 23(4).
van Hooff, Madelon L.M., & Sabine A.E. Geurts (2015). “Need satisfaction and employees’ recovery state at work: A daily diary study.” Journal of Occupational Health Psychology, 20(3), 377–387.
van Woerkom, Marianne, et al. (2015). “Strengths Use and Work Engagement: A Weekly Diary Study.” European Journal of Work and Organizational Psychology, 25(3), 384–397.
Virzi, Robert A. (1992). “Refining the Test Phase of Usability Evaluation: How Many Subjects Is Enough?” Human Factors, 34(4), 457-468.
Yocco, Victor (2017). “Filling up Your Tank, or How to Justify User Research Sample Size and Data.” Smashing Magazine.
Zhang, Zhirun, et al. (2022). “A Diary Study of Social Explanations for Recommendations in Daily Life.” Adjunct Proceedings of the 30th ACM Conference on User Modeling, Adaptation and Personalization, July 4–7, 2022, Barcelona, Spain.
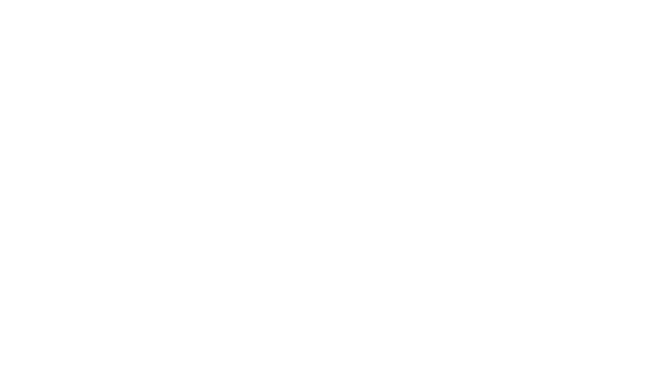